Gathering Diablo 2 Resurrected Gem Data Object Oriented Programming in Python for Yolo v5 (part 2)
- Jason Ismail
- Mar 20, 2022
- 4 min read
This is a continuation of the project found in my part 1 post found here: Part 1
In my last post I had built a Tesseract OCR to read Diablo 2 text from images. The results were good but left some room for improvement. In this part of my project I achieve two things. First I build a place to store the data I have gathered using object oriented programming. Second I greatly optimize and improve my OCR process.
I rewrote my entire project from scratch while implementing Object Oriented Programming in Python 3.9.
Framing my solution in terms of real world assets gave me the inspiration for my structure for the project.
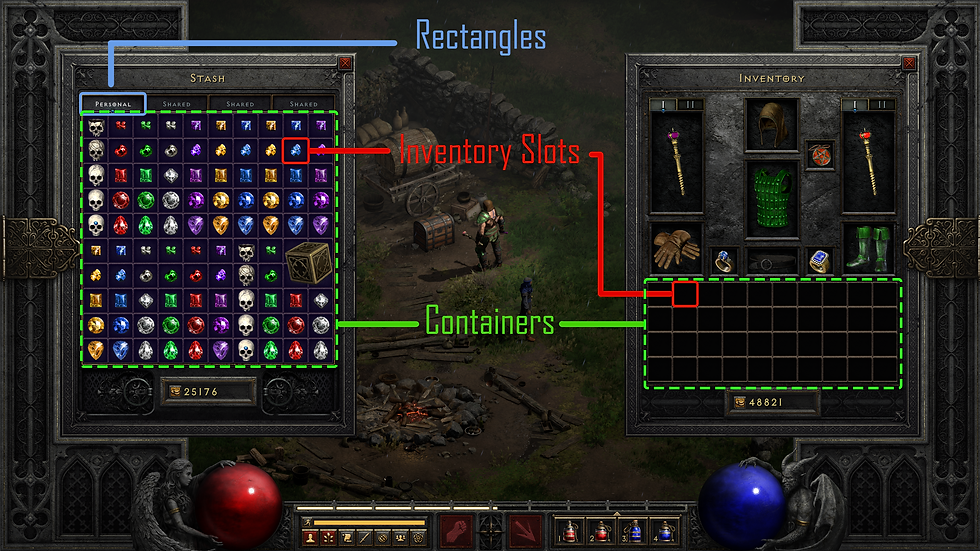
I start by building containers for the players personal stash as well as shared storage. These have similar dimensions and ultimately will hold 100 slots of items. I also build containers for the players inventory and the Horadric cube. All of this is done in a factory class which uses my classes as blueprints to build objects in memory.
Here are some notable classes I built for my project. Each class is defined as a separate python file then loaded in as a custom class library for my project.
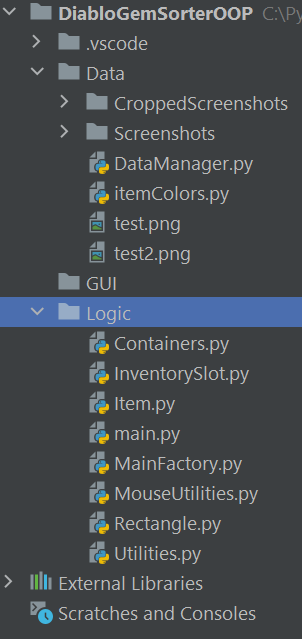
Main Factory: Initializes all containers and Inventory slots.
(Note the tabs here indicate the general structure of the project.)
Containers: Used to store Inventory Slots.
Inventory Slots: Used to hold and keep track of items.
Items: Hold Tesseract OCR text that I extract from images.
(this class is used in many different areas of my project)
Rectangle: Used to define dimensions that are interesting within an image. For instance I use the rectangle class to control areas that can be clicked to switch storage tabs. I use rectangles to crop images of item descriptions for my tesseract OCR. The rectangles will also be used when I build my training data for the yolo network. Yolo requires images with text files that include location data.
Items: Are built to hold my OCR data like item names and descriptions.
(These will be used later to train my Yolo network.)
I using my factory I now have access to all of my prebuilt containers.

The picture below shows my personal stash container. The access location rectangle provides information that I would need for a mouse click for switching tabs in my inventory. My Yolo network will be trained on all slots even empty ones which means I have a bit of unavoidable overhead for my project.
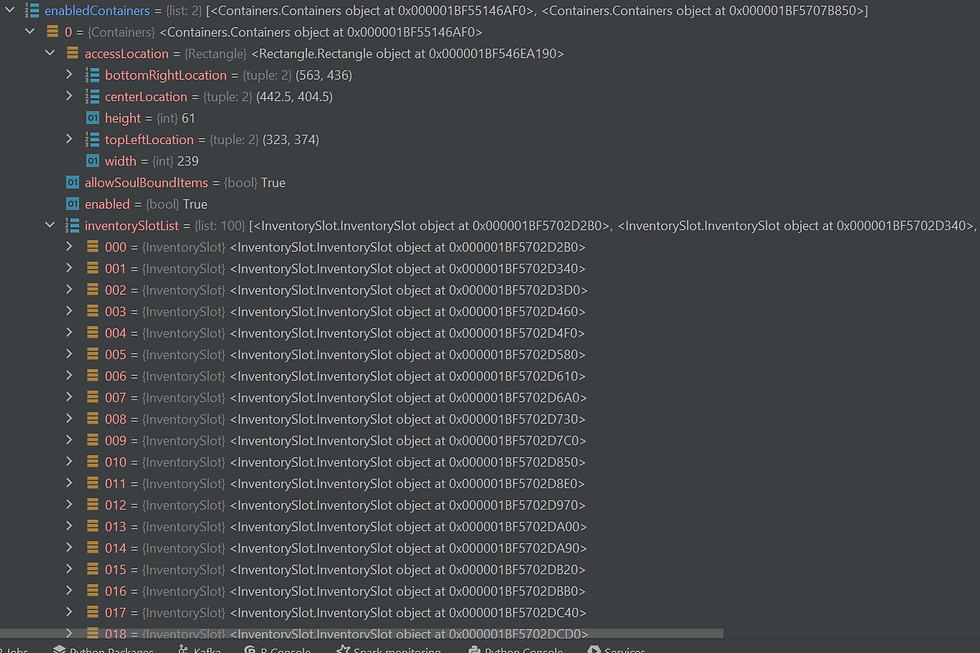
Here I show the structure of the items that I have collected. Each item is its own object so that object can be easily moved to a new inventory slot as needed. You can see the name CHIPPED SKULL and CHIPPED RUBY stored in memory. This will be my YOLO classes that I will be training on. I know their locations due to the fact that they are stored in an inventory slot that has a rectangle which stores location information about the screen.
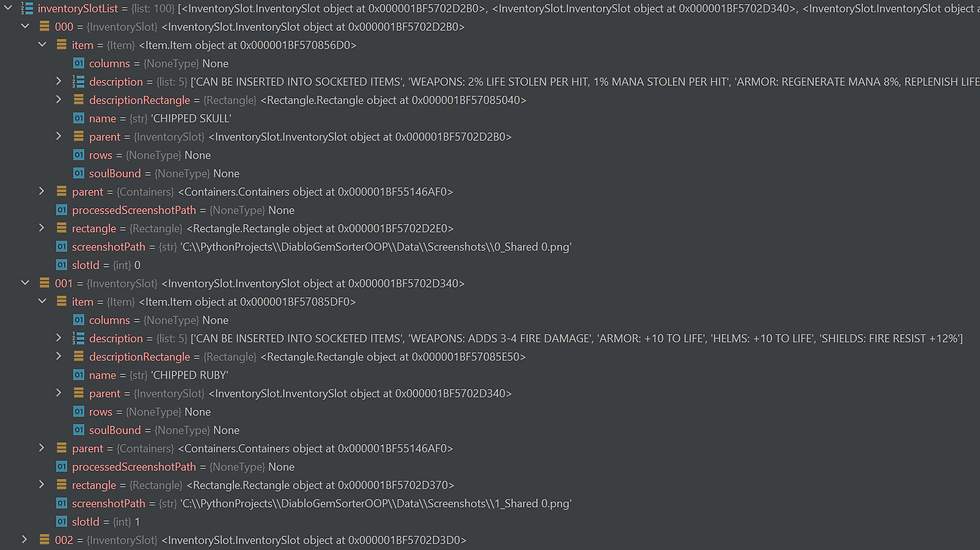
Optimizing the data that I am sending to my OCR.
In part one of this project, I had trained my Tesseract OCR which was the proof of concept for this project. Now I needed to clean up the data that I was feeding to the OCR. Originally I was passing an entire screenshot to my OCR. This increased processing time as well as introduced regions of the screen that could give data that I was either uninterested in or simply generated false results.
Recall from part 1 my results looked like this and the example below is a cropped portion of the screen. The OCR was managing the entire image at a 4k resolution (not shown).
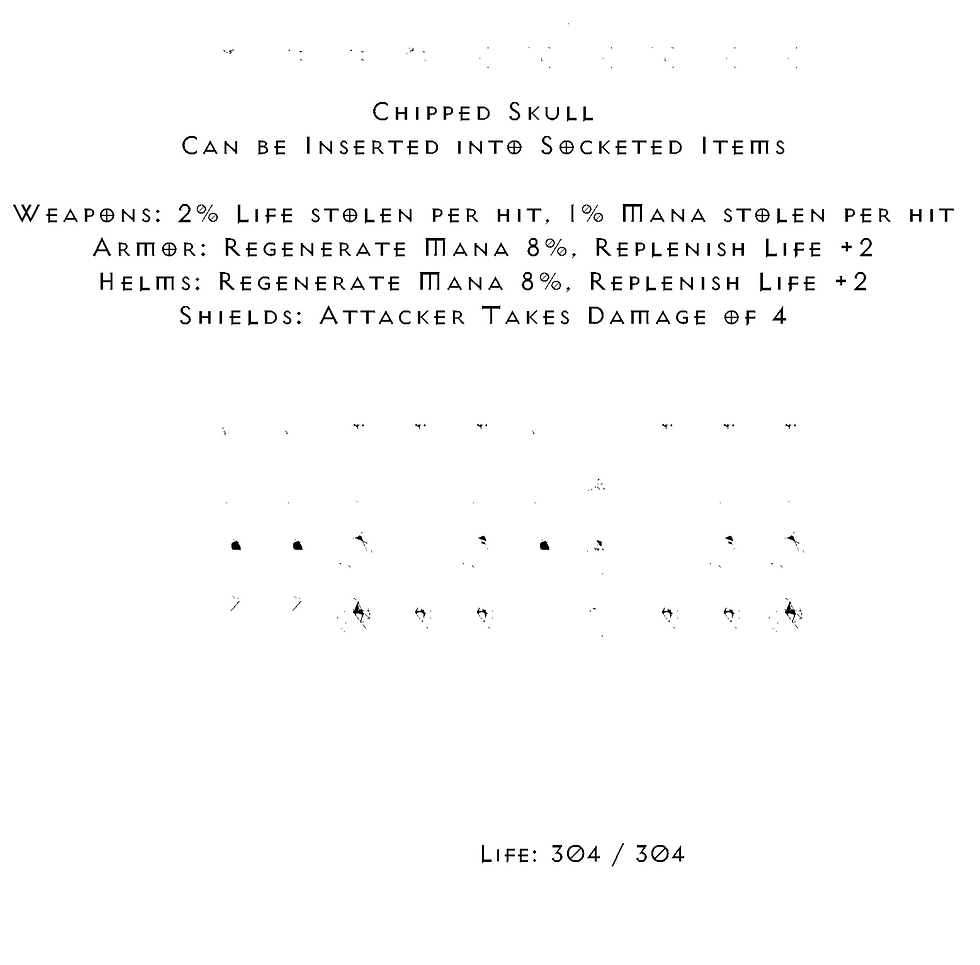
The small black dots generated text that was incorrect. Also other words ended up in the final OCR results that had nothing to do with the item for example Life: 304 / 304.
I fixed this by calculating the rectangle around the items text using a NumPy where function and cropping out the desired pixels. I start at the current cursor location and I alternate moving up and down the screen until I find the description box. To save processing time once the box is found I stop looking in the other direction. I also don't continue looking past the opposite side of the item box.
Results of the python code I wrote to crop the image.
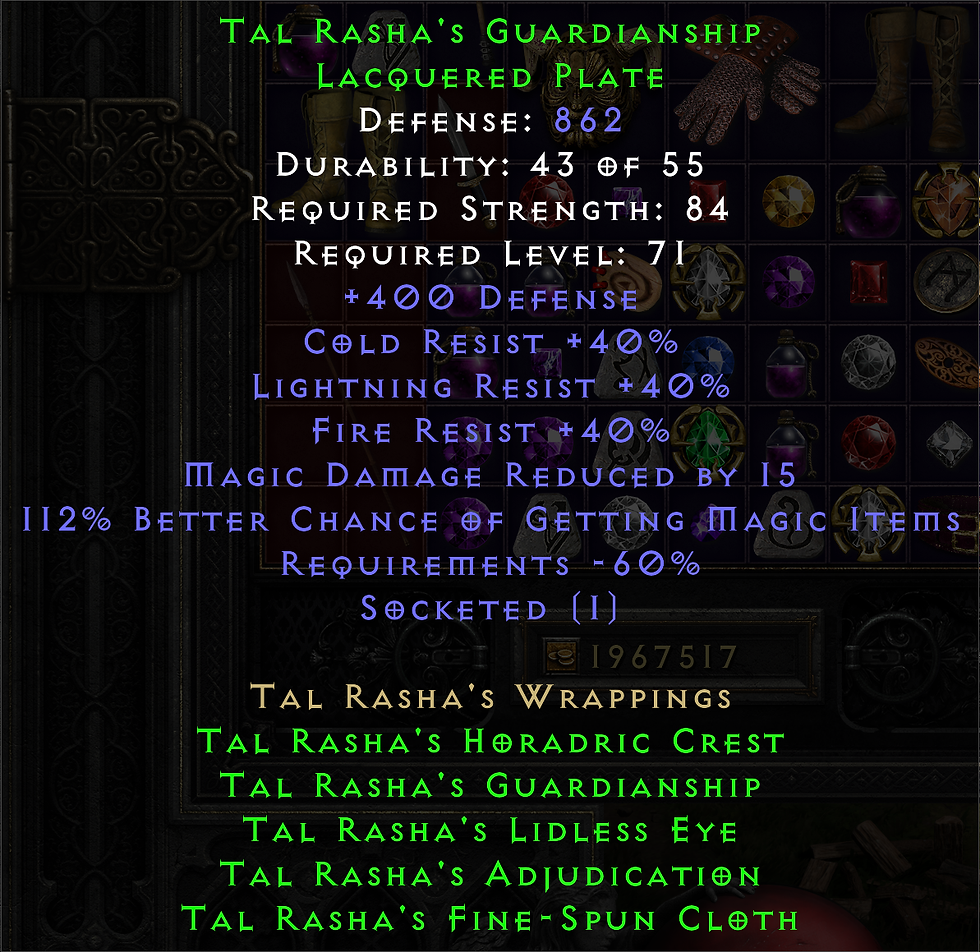
Then I manipulated the image using NumPy to remove colors that I did not want. You can see that I have removed the background so that my OCR does not get confused.
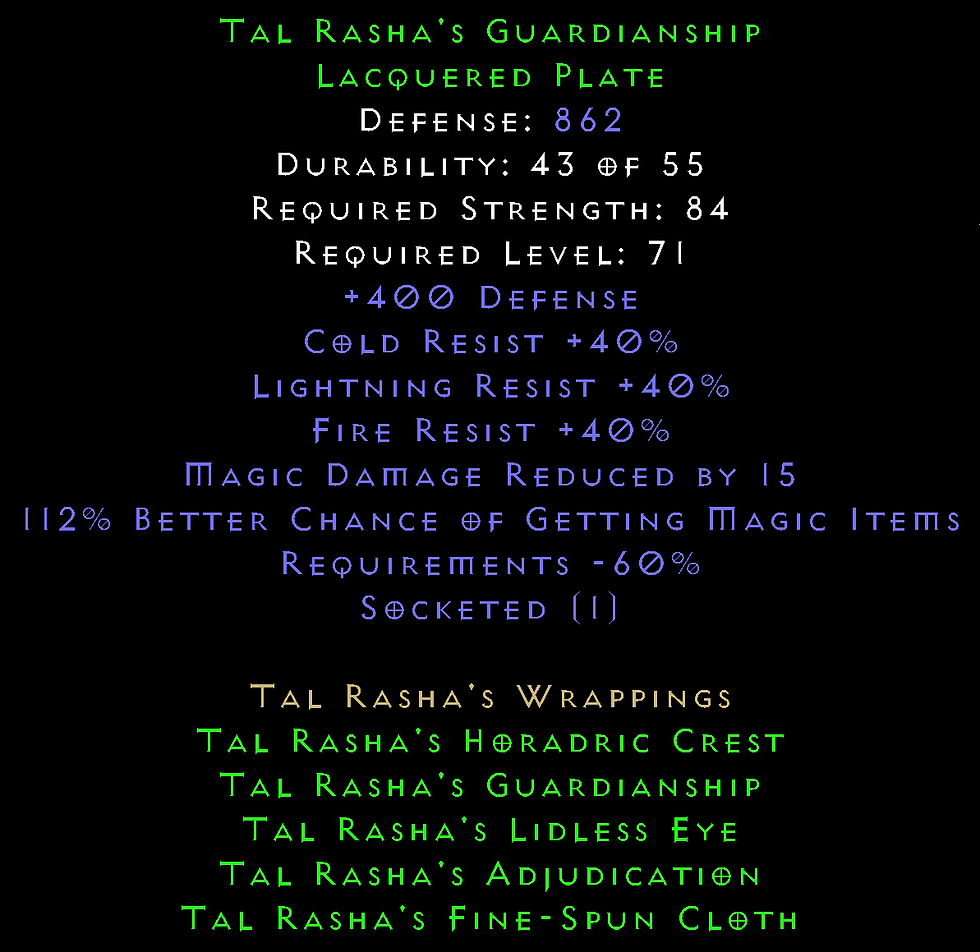
I have opted not to save images to disk and am working in memory now. After a bit of cleanup the image below is what my OCR reads to build my item.

Allowing me to capture the data needed for my Yolo Network. You can see below the name of my stored item is Tal Rasha's Guardianship.
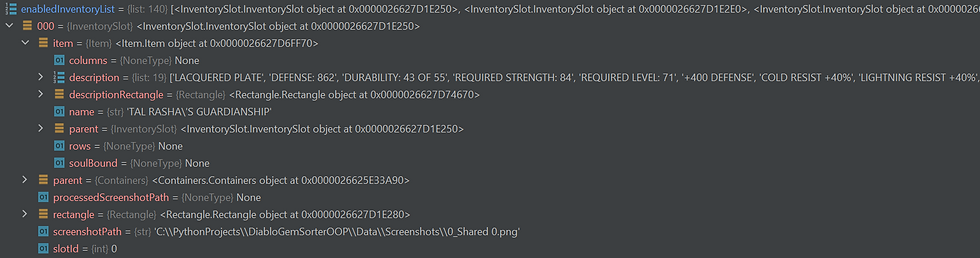
The next step for my project will be building the training files for the Yolo Network using the data that I have collected and cleaned. Stay tuned for part 3.
Comments